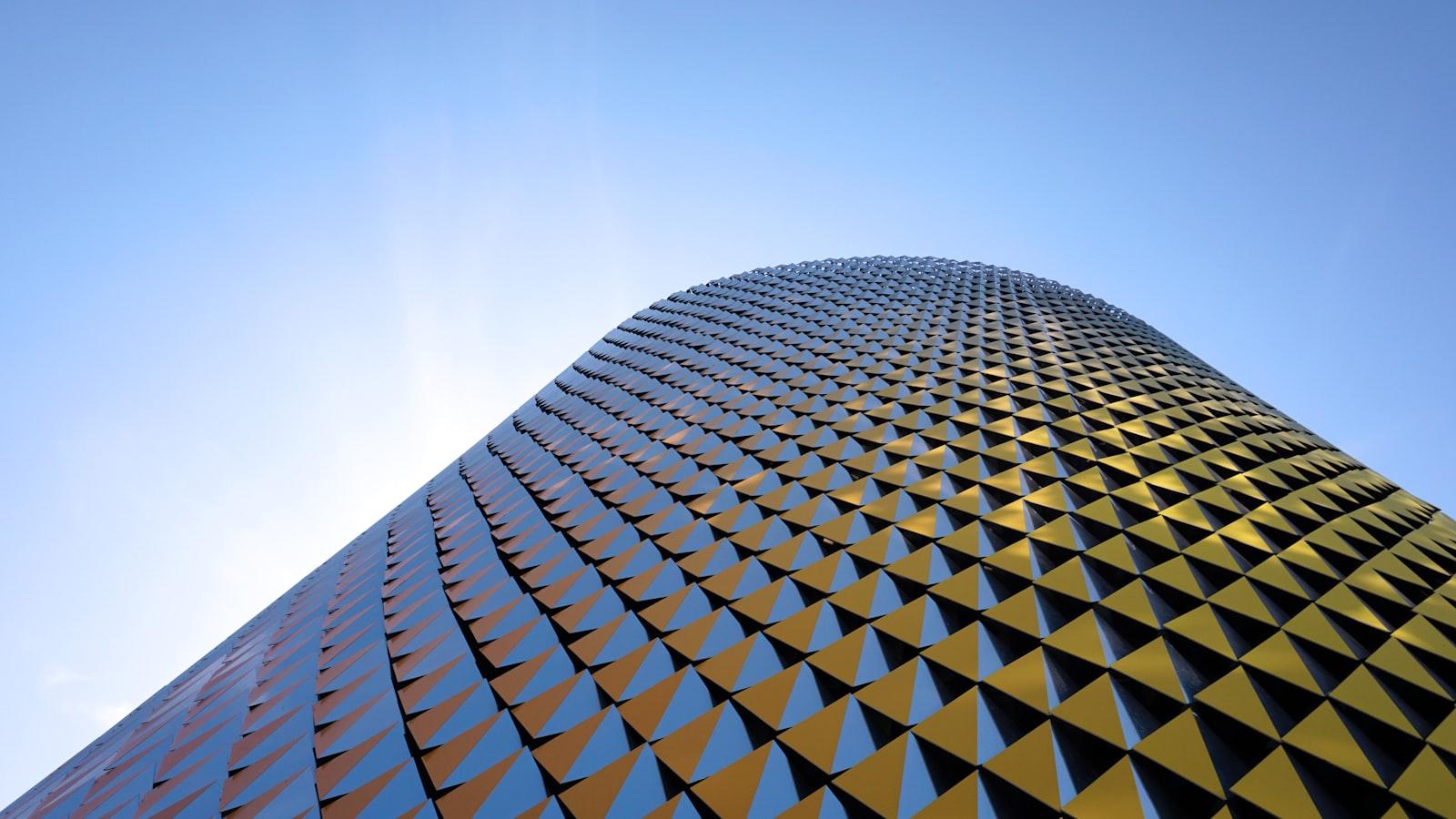
## The Insatiable Appetite: AI’s Voracious Data Demands
The Insatiable Appetite: AI’s Voracious Data Demands
As artificial intelligence systems become more advanced and capable, their hunger for data grows insatiable. These systems require vast amounts of data to train and improve, fueling a race among tech giants and startups alike to amass as much data as possible.
The data demands of AI are staggering, with some of the largest language models consuming the equivalent of millions of books during training. This voracious appetite extends beyond text data, as AI systems also require massive datasets of images, videos, and other forms of data to learn and improve.
The race to collect data has raised concerns about privacy, bias, and the potential for data monopolies. Companies with access to vast troves of user data may gain an unfair advantage, while marginalized communities risk being underrepresented or misrepresented in the data used to train AI systems.
As AI continues to advance, its insatiable appetite for data shows no signs of slowing down, raising important questions about the ethical and responsible collection, use, and governance of data in the age of artificial intelligence.
## Ethical Boundaries: Navigating the Minefield of Data Privacy
The ethical boundaries of data privacy are a complex and ever-evolving issue as companies race to amass data for artificial intelligence (A.I.) development. Here are four key takeaways on navigating this minefield:
1. Consent and Transparency: Obtaining explicit and informed consent from individuals for data collection and usage is crucial. Companies must be transparent about their data practices, allowing individuals to make informed decisions about sharing their personal information.
2. Data Minimization and Purpose Limitation: Companies should collect and process only the data necessary for the stated purposes, adhering to the principles of data minimization and purpose limitation. Excessive data collection or repurposing data without consent raises ethical concerns.
3. Privacy by Design and Default: Privacy considerations should be embedded into the design and development of A.I. systems from the outset. Privacy-enhancing technologies, such as differential privacy and federated learning, can help mitigate privacy risks.
4. Accountability and Governance: Robust governance frameworks, including independent oversight and auditing mechanisms, are essential to ensure companies are held accountable for their data practices. Clear policies, ethical guidelines, and enforcement measures should be in place to protect individual privacy rights.
Navigating the ethical boundaries of data privacy requires a delicate balance between innovation and individual rights. Companies must prioritize ethical data practices, transparency, and accountability to build trust and maintain public confidence in the development of A.I. technologies.
## Strategic Alliances: Forging Collaborative Ecosystems
In today’s data-driven landscape, the race to amass data for artificial intelligence (A.I.) has become a strategic imperative for companies across industries. As the demand for A.I. solutions continues to soar, organizations are forging strategic alliances and collaborative ecosystems to gain a competitive edge. Here are four key takeaways on this pivotal trend:
1. Data Sharing Partnerships: Companies are forming data-sharing partnerships to pool their collective data resources, enabling them to train more robust and accurate A.I. models. These alliances not only provide access to larger datasets but also facilitate cross-pollination of domain expertise and best practices.
2. Open Data Initiatives: Recognizing the power of open data, industry consortiums and government agencies are launching initiatives to make datasets publicly available. These open data repositories serve as valuable resources for researchers, startups, and enterprises alike, fostering innovation and democratizing access to A.I. capabilities.
3. Ecosystem Collaborations: Beyond data sharing, companies are collaborating within broader ecosystems to co-develop A.I. solutions, integrate complementary technologies, and address complex challenges that span multiple domains. These collaborative ecosystems leverage the collective strengths and expertise of diverse stakeholders, accelerating innovation and driving industry-wide transformation.
4. Regulatory Frameworks: As the importance of data and A.I. grows, governments and regulatory bodies are actively developing frameworks to govern data sharing, privacy, and ethical considerations. These frameworks aim to strike a balance between fostering innovation and protecting individual rights, ensuring responsible and trustworthy A.I. development.
In the race to amass data for A.I., strategic alliances and collaborative ecosystems have emerged as critical enablers, reshaping the competitive landscape and unlocking new frontiers of innovation. By leveraging the power of collective intelligence and shared resources, organizations are poised to navigate the complexities of the data-driven era and unlock the full potential of artificial intelligence.
## Regulatory Landscape: Striking a Balance between Innovation and Oversight
The regulatory landscape surrounding artificial intelligence (AI) and data collection is a complex and ever-evolving terrain. As companies race to amass vast troves of data to fuel their AI endeavors, policymakers grapple with the challenge of striking a delicate balance between fostering innovation and ensuring proper oversight.
In this section, we delve into the intricate web of regulations, guidelines, and ethical considerations that shape the data-driven AI landscape. From data privacy and security concerns to algorithmic bias and transparency issues, we explore the multifaceted challenges that regulators and industry players must navigate.
Navigating this intricate landscape requires a nuanced approach, one that encourages responsible innovation while safeguarding fundamental rights and upholding ethical principles. By examining the current regulatory frameworks, emerging trends, and potential future developments, we aim to shed light on the critical issues at the intersection of AI, data, and governance.
## Democratizing Data: Empowering Diverse Voices and Perspectives
The race to amass data for A.I. systems has raised concerns about the lack of diversity and representation in the data being collected. As tech giants and companies compete to gather vast amounts of data, there is a risk that the perspectives and experiences of marginalized communities may be overlooked or underrepresented. This could lead to biased and potentially harmful outcomes, as A.I. models trained on homogeneous data may perpetuate existing societal biases and fail to account for the unique needs and perspectives of diverse populations.
To truly democratize data and empower diverse voices, it is crucial to actively seek out and include data from underrepresented groups. This involves engaging with communities, building trust, and creating inclusive and ethical data collection practices that respect privacy and autonomy. By embracing diversity in data, we can ensure that A.I. systems are more equitable, inclusive, and reflective of the rich tapestry of human experiences.
Final thoughts
As the race to amass data for A.I. intensifies, the future remains shrouded in uncertainty. Will we harness this power for the betterment of humanity, or will it become a double-edged sword that cuts through our very existence? Only time will tell, but one thing is certain – the data deluge has only just begun, and we must navigate these uncharted waters with wisdom and caution.